Have you ever heard the saying: “Not my circus, not my monkeys”? I use it all the time, yet never realized that it is translated from Polish.
Dr. Lynne Bowker, professor of translation technologies and information systems at the University of Ottawa, recently returned from Poland, where she was collaboratively researching ways to make academia more language inclusive. Scholars from around the world are encouraged to publish and attend conferences in English, yet fewer than 10% of the researchers in the world are Anglophones. In fact, for many it’s their second, third, or even fourth language.
Imagine being a world expert in a subject but unable to share your findings and learn from others unless you speak English. With pressing global issues such as food insecurity, global warming, refugees, war, and more, Dr. Bowker set out to find ways in which AI translation or Machine Translation (MT) could help.
Dr. Bowker specializes in language technology. In her recent book, “Demystifying Translation,” she explains why AI and MT translation are today not yet able to solve the issues related to cross-lingual communication – there are limits to what the technology can do and it’s not trustworthy.
The biggest challenges facing authors in academia are the ability to:
-
Read others’ writings.
-
Write in English.
Leveraging AI/MT varies for each of these situations. To tackle the first challenge, the researcher can use automated translation to get the “gist” of what an article says and, with their specialized knowledge, do a pretty good job of figuring out its meaning.
In the second scenario, non-English speaking researchers typically don’t possess English writing skills strong enough to clearly write their findings. This means that they would need to pay someone to write it or have a highly skilled translator edit their original draft. To simplify access to research, the academic community must get to the point of accepting publications in a researcher’s native language, with readers relying on AI/MT for gist translations. Lacking this option, many researchers will continue to opt out of publishing or presenting at conferences to share important research.
At the same time, Dr. Bowker stresses the importance of recognizing that language technology is not perfect and ultimately requires nuanced discussion and experienced judgment regarding where and how to use it. It’s not merely a discussion as to whether AI/MT will replace humans in translation.
In fact, automated machine technology is old tech. During World War II, experts developed tools to decipher what the enemy was saying – spying tools, essentially. The technology relied on dictionaries and linguistic rules for grammar to translate, and had particular trouble with synonyms (two meanings for one word) and polysemes (words with more than one meaning – for example “pen,” as in a writing pen or play pen). Ultimately, the technology was used only by professional translators because it was not good enough to release.
Throughout the ‘80s and ‘90s, the technology in general improved – it became faster, smaller, and required less storage. Programmers started thinking of new ways to process language – by using data-driven approaches instead of linguistic rules. Translation quality improved in general but still fell far short of high quality.
Current advances have changed from data-driven analysis to pattern identification, whereby machine language learning develops a memory by ingesting vast amounts of multilingual text. Without the large amounts of data, the technology is useless.
Dr. Bowker recalls a time when Canadian weather forecasters struggled to hire English-French translators because the work was so repetitive – the words and format were always the same. After a few months, even new undergraduate translators quit from boredom. By leveraging AI/MT, the organization could save time, money, and effort.
Technology still struggles with:
-
Languages that produce less content – languages like Welsh and Danish have fewer speakers, thus less content is produced. It’s challenging enough to develop a machine learning (ML) model that can translate from English to Welsh, let alone from Welsh to Danish.
-
Unusual topics – in research, topics are usually novel and don’t have a gathered history of translation. This challenges the technology to find appropriate translations.
-
Hallucinations – one team set out to test whether AI could properly classify an animal as a huskie or a wolf. After training the model on hundreds of pictures, its responses were mostly accurate; however, upon further inspection the researchers figured out that the model had been trained to identify wolves by snowy backgrounds, versus huskies in backyards. Thus, the model looked at the wrong information. Training for proper identification of skin lesions produced the same outcome – the model identified cancerous lesions by looking for a ruler in the picture.
-
Misogyny and racism – models learn and parrot back the information ingested and, simply put, the Internet provides lots of questionable content
The bottom line, according to Dr. Bowker, is that while we are still far away from a world in which automated translation can replace human translation, the language industry will only benefit from educating people on proper and effective translation methods, in the meantime.
When translating your material, remember the iron triangle (aka project management triangle), which illustrates how the balance among three project constraints – scope, time, and budget – affects quality. Adjusting for any one constraint necessarily affects one or both of the other variables:
-
Fast and cheap = lower quality
-
Fast and quality = very expensive
-
High quality = pricey and slow
Finally, we agree that the industry conversation will turn toward the increasing need for experts in global content strategy. Think about the content along your buyer’s (or stakeholder’s) journey – from first encounter to raving fan. A thoughtfully planned strategy alongside clearly defined processes and technologies will guarantee high-quality content for every audience, while saving your employees time and effort.
Links:
Book: De-mystifying Translation: Introducing Translation to Non-translators
With a Creative Commons license (CC BY-NC-SA)
LinkedIn: https://www.linkedin.com/in/lynne-bowker-0112996/?originalSubdomain=ca
Email: lbowker@uottawa.ca
Connect with Wendy : https://www.linkedin.com/in/wendypease/
Music : Fiddle-De-Dee by Shane Ivers https://www.silvermansound.com
ATTENTION: Below is a machine-generated transcription of the podcast. Yes, here at Rapport International we talk a lot about how machine translation lacks quality. Here you see an example of what a machine can do in your own language. This transcription is provided as a gist and to give time indicators to find a topic of interest.
Wendy: Hola amigos and welcome to another episode of The Global Marketing Show. As you know, or maybe you don't, we're brought to you by Rapport International, the company that connects you to anyone around the world across 200 languages and cultures by providing high-quality written translation and spoken interpretation services.
[00:01:00] And we have a tidbit from Rapport International today and that's the Polish expression, you might have used it. It's one of my favorites. It's not my circus, not my monkeys. When you're talking about something you don't need to get involved in and this ties into today's episode. So I'm looking forward to telling you about that.
Speaking of today's episode, we are going to talk to Dr. Lynne Bowker. She's a professor at the University of Ottawa and her area of specialty is language technology, so you know all that AI translation and Google Translate and can you use it, where does it fit in, well this is her specialty and she actually just published a book called Demystifying Translation and she's given us a link to a free download if you'd like to download it and learn more, we'll put that in the show notes so you can find it there.
And Dr. Bowker has just returned from [00:02:00] living six months in Poland. So Dr. Bowker, I'd like to welcome you.
Lynne Bowker: Thank you so much for the warm welcome. It's really a pleasure to be here. I'm recently back in Canada after my Polish adventure. And yeah, it's really good to be here and, chatting with you today.
Wendy: Okay, so I had that Polish expression, not my circus, not my monkeys, which we actually talked about before, and you can say it in Polish. Can you tell me how to say it in Polish?
Lynne Bowker: Okay, my accent's not wonderful, but nie mój cyrk, nie moje małpy.
Wendy: That sounded great. I'm going to have to listen to the recording again and again so I can say that when I want to say not my circus, not my monkeys.
So what did you think of Poland?
Lynne Bowker: It was an adventure. I did not really know what to expect before I headed over there and it was really a wonderful experience. Very warm
and welcoming people, [00:03:00] challenging language. But people appreciate when you make the effort, they really do. And, you know, very easy to do things, technologically over there.
Many different kiosks offering options for, you know, grocery shopping, buying bus tickets, everything that you need in your daily life. If you really are struggling with the Polish language, you can often buy them through an automated kiosk, which offers, the options of English, sometimes also German, Russian, other languages. So really easy to get around. But also just really friendly and, a new cultural experience for me. I've spent more time in Western Europe. My working languages as a translator are French and Spanish. So I've kind of, as you know, spent time in those countries.
So it was really a big adventure. I registered for a language and culture class. So that was fun to just to learn a little bit about, I mean, I'm nowhere near fluent, of course, learning a language takes a lot more than a few months, but it was still a great opportunity to have some insights into language and culture in [00:04:00] Poland.
Wendy: That's fantastic. And what were you doing over there?
Lynne Bowker: I was on sabbatical leave from my position at the University of Ottawa, and I was working on a research project at the Adam Mickiewicz University in Poznań, which is in sort of the Northwest corner of Poland. And I was working on a project on machine translation and using machine translation in a scholarly communication context.
English has kind of bulldozed the landscape of scholarly communication. So academic publishing sharing research findings; everyone around the world is pressured to do that in English now. And while there are some benefits to having a common language there are real disadvantages for people for whom that's not their native language.
And if you think about someone who has spent time becoming a top quality researcher in a field like physics or law, why should they also be speakers of English, you know, [00:05:00] like they've invested so much of their time and energy in becoming an expert in their field. And then all of a sudden they're told, well, yeah, but if you don't speak English, and if you don't share your research results in English, nobody's going to publish them.
So we were looking at how to make the scholarly communication ecosystem, the environment of scholarly communication, more multilingual. and where technology might be able to help with that. So, anything from publishing an
article in another language, or offering subtitles maybe for conferences for people, because the conferences too are often held only in English, and for some people who are not comfortable speaking in that, in their second language, like, it's very anxiety-inducing, and they would often just opt not to go.
And we can't afford that. We have so many problems in the world right now. Climate change, food insecurity, COVID, cancer, all of these things. We [00:06:00] need the best brains on the whole planet working on these problems. And we don't want to be excluding people because their knowledge of English is not good enough.
you know, considered high enough or good enough to publish or to present in English. So we, we need to get away from, from this. Like I said, it was just like a bulldozer of English kind of pushing all the other languages out of the way. And that's not good for science. It's not good for society. So we need to kind of find ways of making this ecosystem more multilingual again.
And I think there's a role there for technology.
Wendy: Yeah. Yeah. And we're going to get into that. It's so interesting because I have talked to so many companies in my role at Rapport International about their troubles of hiring good people to work in manufacturing or other highly skilled areas where people might be good at doing that, but they might not speak English so they can't get a decent job in the United States. And [00:07:00] how do you adapt the workplace to be more inclusive across language? But I had not even considered that in the scholarly area, which my parents were an international research and professor, so it's so interesting to hear.
Is machine translation going to be able to bridge that gap?
Lynne Bowker: So I think there's a role for machine translation, but I think we need to approach it in a sort of nuanced way, right? It's not a panacea, it's not going to solve all the problems. It's not a perfect technology, but it certainly can help.
There can be a role for it. And our challenge at the moment is understanding that role, defining that role, you know, what are the limits, what things should we perhaps not be trusting to machine translation and where can we be a little more confident that machine translation is the right route?
So, yeah, it's that sort of judgment that we're trying to develop now around the smart use of this technology.
Wendy: And what are your preliminary findings? Like where would you see the uses [00:08:00] and not uses?
Lynne Bowker: So, if we go back to the scholarly community example just for a starting point, we could say that machine translation could be used in two very broad ways.
One, to help us understand things that have been written in a language that's not our own, that we don't understand very well, or to help us write things in another language, right? So on the one hand, using it to help us assimilate knowledge, help us understand things, and on the other hand, to help us produce knowledge and share things.
And machine translation is probably much safer and smarter to use for the first one of those tasks. Because even if we don't understand the language that the text is written in, and so we translate it using a machine into our own language, if we're a subject expert, we can kind of spot what might not sound right, where there might be a mistake, because we know the field so well, we know the subject matter, and so if there's something that seems a bit [00:09:00] suspicious and weird, we can say, well, that might be a translation error, so I can flag that, and I can, you know, double check that in another resource or something. When we're trying to say for the majority of scholars who are not anglophones, but who are being pressured to publish in English, if you were, for example, a Polish speaking scholar, and you wanted to publish in English, but your English isn't very strong, how can you really assess the quality of the English text that's been produced with machine translation?
You can't. So that means you either have to pay someone or hire someone to do that for you. So, that is sort of a less perhaps good use of machine translation unless you have that second step where someone can check it for you and verify it for you. And so that creates another barrier for non-English speaking scholars because now all of a sudden they got to pay people to translate their work or edit or verify their work.
And many of the non-English speaking scholars are probably not in the most wealthy regions either. So if you think [00:10:00] about, well, the whole of South America or Africa, some of those scholars would be really struggling to come up with the money needed to have somebody translate or edit or verify all of their work that would be produced in English. So what we'd like to do is get to a state where people can be comfortable publishing in their own language. And then machine translation is a great tool, as I said, for helping readers who translate it into their own dominant language and who are subject experts and who can therefore kind of verify for themselves, whether something seems to be legitimate or not, whether there are problems.
So that's one example of how it's not sort of a blanket like machine translations, good or bad. It's sort of like it has good applications and less good applications. So the whole discussion, it needs to be nuanced, I would say.
Wendy: Hey, right. It is so nuanced because even in the second situation that you're talking about, [00:11:00] that they write in another language, they use machine translation to translate, then they would need a reviewer that would have the language skills and the subject matter expertise, which is really hard and really expensive to find.
Lynne Bowker: Absolutely. Yes. And in our current climate where we're sort of insisting, you know, as the scholarly community as currently sort of insisting that everyone publish in English, fewer than 10 percent of the top researchers in the world are anglophones.
So it really sets up a very uneven playing field where people who have to work in their non dominant language, because it's not even only a second language. For some people, it might be their third or fourth language, English. You know, they are having to invest so much more time. I mean, if you've ever tried to do anything in another language, you know that it takes a lot more time and money as well.
Well, as you say, it needs to be edited, it needs to be [00:12:00] fact-checked by somebody else, and that is not cheap when you're getting into those kinds of specialized knowledge that's needed for that. So there are a number of disadvantages. And now we're talking just about publications and there's a whole other side of scholarly communication, which is conferences, presentations.
And that too is very challenging for people who are being asked to do that in a non-dominant language. Some people just opt out. They're like, it's so anxiety-inducing for some people that they just sort of say, no, I'm just not going to do it. And then the whole world misses out.
Right. So what do you do about that? Machine translation technology is also being integrated into kind of speech recognition tools. And so we're getting tools that will offer what we might describe as automatic interpreting. So the tools are able to hear spoken language input and then translate it and then either show it as subtitles often or maybe with speech generation subtitles a little more [00:13:00] common at the moment.
And you might have seen that if we're doing online conferences on Zoom; we often have the option of, putting on captions. And if we link that to a machine
translation system, we can get the captions in other languages as well. So for online conferences or even an in-person conference, you can still have a screen where you can project that, or an app that people can have on their phone or their tablet.
So the possibilities are kind of exciting. I would say it's an exciting time. To be working, but we always do need to recognize that the technology isn't perfect and we need to not be on sort of autopilot where we're just accepting everything that we see out of a machine translation system at face value.
We do need to bring some good judgment to the table because the technology is not perfect. That doesn't mean it's useless, right? And that's the problem we see in the press, the general language press now, not scientific press, but just newspapers and social media. We often see a very binary and polarized [00:14:00] discussion of machine translation where some people are really hyping it up, like it's, you know, just the best thing ever.
And it's superhuman because it is fast and it is convenient, but people sometimes ascribe too much power to it because it does have some problems. So we have all the hype on one side. And then on the other side, we often have the really extreme negative views, which are because it's not perfect, it's completely useless.
And of course, the truth is somewhere in the middle. So that's why I just keep emphasizing this need for more nuanced discussions everywhere around machine translation. It's not a binary thing. It's not good or bad. It's good for some purposes, poor for some other applications. So we're learning as we go what these good applications are and what the less good applications are.
And some of it's by trial and error, and some of it could be more predictable than others. But what we really are in this phase of learning and developing our judgment, [00:15:00] and of course, in the translation community, we've been dealing with technology for a while.
It's actually quite old technology. It dates back to the first days of computing, which were in the 1940s. And we often think about translation in a very positive way as building bridges and breaking down barriers. But unfortunately, the origin story of machine translation is set in war time and spying, and kind of trying to figure out what the other side was saying.
So it was in this period just after the Second World War that machine translation tools were first developed. But at that time, because computers were so new, we didn't really know a lot about computing. They approached the
challenge of machine translation as a linguistic challenge, which of course is a quite logical place to start because, translation between humans is based on a lot on linguistics.
So they tried to develop these tools by programming grammar rules into them and building very large dictionaries for them. And this only took them [00:16:00] so far because if you think about, about people and how people communicate, yes, we use grammar. Yes, we have vocabulary. But we also use common sense, we use our knowledge of how the world works, and that is something that computers don't have.
They just don't understand anything, and they don't have any kind of ability to apply just this logical understanding of the world. So we have lots of examples in all languages of synonymy, where two words can mean the same thing. Or something which is called polysemy, where there's one word, but it can describe two different objects or two different things.
So a word like pen, for example it could be something that you write with a pen, or it could be a place where a baby could sleep or a place where an animal could be kind of contained. So, if I said to you, put the baby in the pen, [00:17:00] you would not even think about trying to cram a baby into a writing instrument.
Like that just wouldn't even cross your mind, right? Because it's just like this kind of world knowledge and common sense. We have it and we use that so often. in communication. And that is something that computers don't have. So for the computer, it's like a 50 50, right? Would I translate it pen as in the writing instrument or pen as in play pen.
And so they tried very hard to build kind of cues and linguistic issues to resolve these problems. But it really was not successful because, as I said, you can't give computers common sense. You can't give them world knowledge. And so for many years, for the first 50, 60 years that machine translation was around, it was really only used by professional translators because they were able to compensate and fix the problems in the output.
And it wasn't really safe, I guess the quality wasn't good enough to release [00:18:00] it to a broader public who didn't have a translation background. So translation tools stayed in the hands of translators for many years. And it wasn't until the whole of, I would say, like the sort of technological landscape began changing in the 1980s and 1990s, we saw that technology was faster, smaller, had more storage power, right?
Just the computer technology advanced a lot. And we started getting desktop computers on everybody's desk in the 1980s. And now a couple decades later, we have computers on our wrist and in our pocket all over the place. So technology advanced. So that was one important thing.
Something else that happened was that because people had computers on their desktop, we started producing texts in electronic form. We didn't handwrite everything and send it to the typing pool anymore, we word processed it. So now all of a sudden we have a text in an electronic form that is machine [00:19:00] readable.
So that was a second really important change that happened in the 80s and 90s. And then a third one was the internet. It became really easy to share information over the network. So, because we had increased technology and processing power, we had increased availability of text in electronic form, and we had increased ability to kind of share and store that information in places where people could access it.
The computer programmers started to think about new ways of approaching the challenge of machine translation. And ironically, the new ways don't involve a lot of linguistics. So we think of translation as a linguistic activity, but the new approach to translation is a data-driven approach. And basically, how it works is, the developers of the tool gather lots of examples of texts that have been previously translated.
So if you [00:20:00] go to any government of Canada website, for example, you're going to find texts there in English and French, because it's a country that has two official languages, so it's quite easy, you know, if you're going around the world to the World Health Organization or the United Nations, they all have multilingual texts available on their websites.
So if you gather enough of these previously translated texts, you can feed them into the computer. And even though computers are not good at linguistics, and they're not good at common sense, they are good at pattern matching, and they are good at number crunching. So these are the strengths that computers bring to the table, and a data-driven approach to machine translation capitalizes on these strengths.
But you need a huge amount of data, and that's currently the challenge that we're facing, right? It's not enough to have two or three examples. For machine learning, for a computer to learn something, it needs thousands of examples. So we can [00:21:00] put together these, well, they're called corpora, but they're basically text collections of previous translations.
We can put together collections of previous translations in widely used languages quite easily. So English and French are good examples. And as I said, the Canadian government is a fantastic source for this. So we can gather lots and lots of examples of French to English or English to French translations.
No problem. But it gets harder as we try to get corporate for less widely used languages. So a language like Welsh, which has only a few, you know, million speakers, not very many, or a language like Icelandic or a language like Danish, any country that has a relatively small population, there are probably fewer examples of text because they just probably produce less.
And when we try to combine two less widely used languages, the problem gets even harder, because we don't just need to collect [00:22:00] collections of text, we need collections of translated text, we need like the source and the target text in the two languages. So if you want to translate from Welsh to Danish there's probably not very many texts available in that language combination.
And then when you start throwing subject matter at it as well, and you say like, well, I have a text that I want to translate and it's on nuclear physics. Okay, how many Welsh to Danish text translations on nuclear physics are there? And we get down very quickly to to a real challenge. We can't put together a large enough corpus for machine learning.
Machine learning needs a huge amount of data. So we're again in this situation where the technology works well for the languages that are widely used. And they're the languages that don't need the support as much. So we're circling back to this same problem where it's the languages that are not well supported in the first place are now being even further marginalized by this approach to technology, [00:23:00] because it's not the technology, but it's the lack of data.
And then the fact that it's a data-driven tool without the data, it's useless. So we're struggling with this problem of how can we gather relevant data in the less widely used languages and, and the less common subject topics, because if you want to translate medical texts, you can't have a corpus of legal texts.
That's not very helpful, right? It doesn't have the right terminology. It doesn't have the right phraseology in it. So you need to not only take into account language, but also subject matter and sometimes even text type. Some text types have a very peculiar structures. Like if you want to translate a recipe, a cooking recipe, like that's got a very specific kind of style, right?
Of how you format and organize information in a recipe. It's not like a legal contract, like that looks different somehow. So we've got text typology issues,
subject domain issues language issues, language pair issues. [00:24:00] So although the technology is able and can work. We need the data. And that's the problem that we're facing right now.
Wendy: So, I mean, from my standpoint, I see that language changes a lot. I've got a dictionary from the 1930s that's about a quarter of an inch thick. If I pull out my Webster's dictionary that I might have around here somewhere that's like four inches thick. So, there's a lot of words that are added and then every six months the Webster's takes out words and adds words as we add them in. So language is fluid. Do you see this ever taking over for humans?
Lynne Bowker: That's a question that is not best answered with a yes or a no. I think there are some areas where it will take over and other areas where it will not.
And again, I can give like a kind of a fun historical example of machine [00:25:00] translation. One of the most successful ever applications of machine translation was a system that was built to translate weather forecasts. And part of the reason that it was so successful was because, well, weather forecast is a very restricted field, right?
And it was not just weather forecasts. It was translating weather forecasts from English to French for Canada. Canada only has so many types of climate. It doesn't have like a Saharan desert or anything like that. We were limited in the language pair and limited in the text type.
So it was a kind of a perfect storm in a positive way of an area that was a good fit for machine translation. But one of the most important factors was that people don't want to spend their week translating weather forecasts. They couldn't find people. They were getting new graduates who it was their first job and they were desperate and they would have done anything and they would stay for like three months and then as soon as they got [00:26:00] any other job.
They were gone. So it was like a revolving door. They just couldn't keep people in the job. So that's a perfect win-win, right? People don't want to do it anyway, so why not let machines do it? So there are going to be other things like that where it is a good fit, but then there'll be others where it's not a good fit.
And probably what we're going to find is that the best fit of all is a team approach, right? People being supported by these tools. And I think that that will be more and more common in more and more fields. And health is one great example where we probably don't want to trust health related decisions to AI, but AI can help doctors.
It can help them spot patterns that they might not have seen, but then it's up to the doctor to interpret the pattern, right? The machines can show us things and then our, you know, skill is not pattern matching or pattern recognition. That's a computer skill, but our skill is interpreting. What does the pattern mean?[00:27:00]
That's important. And again, I have another funny example if I can just share of one of the early machine learning applications. One of the first things they tried to get it to do was a classification of things. So actually of images, visual things. And they built a system and the way the systems work is you give them some training data.
So you have to start by showing them and telling them what this is. And they wanted to build a system that could look at a photograph of an animal and decide whether it was a husky dog or a wolf. So if you know huskies and wolves, they look a little bit similar, but they wanted to see, could they train a computer system to look at a photograph and then classify it?
Is this a husky or is this a wolf? So they start off by showing it training data. Here's a hundred pictures of huskies. The computer looks at them, look at these pictures. These are all huskies. Learn what a husky looks like. And then they show it a hundred pictures of a wolf and they say, this is a wolf.
Look at these pictures, look [00:28:00] what the wolf looks like. And then they find some new pictures that weren't part of the training data. And they say, okay, here's a picture. What is this? Is this a husky or is this a wolf? And they were shocked that showing it 25 pictures, 50 pictures, 100 pictures. And it was like getting most of them right.
And they're like, this is amazing. We've developed a system that can classify things. But when they started to look at the few examples where it was wrong, because it occasionally got it wrong. And they really tried to analyze what was happening. They realized that the problem was that all of the pictures that they had had in their training data of huskies were huskies against a non-snowy background. And the wolves were all against a snowy background because the wolves were all in the wild and the husky was in your backyard. And what they had actually been training was a snow detector machine.
[00:29:00] It wasn't even looking at the animals. So this is the problem with machine learning. It finds patterns. It's not the pattern that we were expecting or the application that we intended it for. So the huskies and the wolves is a funny example. It's hilarious and who really cares if it makes a mistake, but when they try to apply this in other more serious contexts like health, they tried to do it by showing it pictures of people that had a skin lesion and asking whether or not
that skin lesion, like a mole, for example. Did it look cancerous or not cancerous? Because they thought, this could be a good thing, like as a detecting, right? Can we, can we show a photograph of a mole on someone's arm to an AI and will it be able to see whether it looks like a cancerous mole or not? And again, what happened was all of the pictures that were cancerous, happen to have a ruler in the picture because the doctors often want to measure the size of that [00:30:00] lesion.
So it was not a cancer detector, but a ruler detector. So we really need to, yeah, as I said, we're still in this phase of learning what are safe things to give to a machine and what are not. It's good at pattern matching, but sometimes the pattern it's spotting is not what we had intended it to look at, and it doesn't have any common sense.
That's a legitimate pattern. Is there a ruler or no ruler? Is there snow or no snow? That's a legitimate finding, but it's not a helpful one. It's not what we want. So we have so much work to do in learning about the training data that is useful, right? We have to find the right data.
And if our data is kind of messy or not curated well, then it can find patterns that we don't want it to find. And we've seen some really disastrous things. We've seen really misogynist language and racist language, because if you don't carefully curate [00:31:00] the training data, the machine will learn from what you give it.
So if you're giving the machine texts that are, you know, racist or sexist, it learns that language and it will parrot it back to you because it doesn't have common sense. It can't make a judgment like, Oh, that is not appropriate. So it's an exciting time, but there's a ton of work to do.
We're really far away from saying that machines will, you know, take over. We're so far away. I'm not afraid of that. I do think that we will find great applications for technology language technologies, but we're also gonna find, some areas where we just say, no, that's, that's not right.
Wendy: Okay. As someone who's involved with the language translation company, and you know, Rapport International, we have a hundred percent guarantee. We've always used humans in appropriate cases. We use translation memory when we can leverage that technology. We've done this before.
This is what it was said. We manage it carefully. [00:32:00] Now we're seeing people say, Oh, well, we're going to just put Google Translate up, or we're going to use AI to do this, or it's a lot cheaper to use a machine translation.
There are agencies that are out there that will provide machine translation with post editing, but it still doesn't sound good and natural.
People are going to skim over it. How do you explain all this? That you've just taken us through and like how do we make it sound different, you know, and I'm asking for all of us agencies that are out there. That know all this intuitively because we've worked in the industry.
Lynne Bowker: You've really hit the nail on the head in terms of the problem of educating the broader public about all of this and this is a challenge that I'm struggling with as well. I call it machine translation literacy, because we basically need to inform the public which includes our clients right.
About this need for judgment. And,[00:33:00] we also need to admit as translators that there could be some types of texts that don't need to be super elegant. And the weather reports were a good example, right? And those are texts that we don't want to translate anyway. So there may be kind of wiggle room, right?
And we also have this idea that translators are probably familiar with, although they maybe don't call it the same thing, but this thing called the iron triangle or the project management triangle. It basically says that on a triangle, there's three points quality, cost, and time.
And you can rarely have all three in a favorable kind of format at once, right? It's usually you can get two out of three, and you have to flex on the third one. So you can have something fast and cheap, but it won't be good. You can have something fast and good, but it won't be cheap. And you can have something, yeah, good and cheap, but it won't be fast.
And that is an effective little tool for [00:34:00] showing clients. And machine translation is definitely fast and it's definitely cheap, but it's not as good as a human translator. And so if they want to, if their flex point is quality, then machine translation is a great solution. But if quality is important to them, then they need to keep humans in the loop and flex on one of the other points instead, which means they might need to give a bit more time or they might need to be prepared to pay a bit more. And I think that's reasonable, and this project management triangle is not unique to translation. This is a business model. Every business has to deal with this.
So I think translators just maybe don't talk about it in the way that some other businesses do, right? It's just not as clear. And I think that that project triangle is a great conversation starter. Because then it's clear, it's their choice. Is it really
quality that they want to take the hit on? If that's where they want to take the hit, you probably don't want them as a client anyway.
Wendy: [00:35:00] Yeah, no, it's a standard business thing. When I was in MBA school, we learned about that. Don't try to be everything to anybody. You've got to have that triangle. So that was years ago. And I hadn't put that in perspective here. So thank you for bringing that up.
Lynne Bowker: But I think as people, as translators, our strength is that quality angle, right?
Like, we are not as fast as computers, and we are not as cheap as computers, but we are sure able to bring quality to the table, and we can use computers to help us be a little faster. And maybe that means we can give some kind of discount, but it can't be an abusive discount, and it can't be an impossible deadline.
I think that translators can leverage technology, but that they still are in a position of power because they bring quality to the table and quality is often judging what output of machine translation is salvageable and what isn't. The challenge though is making [00:36:00] people who are not in the translation field understand what is our value add.
And that's where we need to be able to articulate it. And sometimes we have trouble ourselves articulating it. So we need to work on that as a community. Yeah. As a translation community.
Wendy: All right. So now I got another question for you with all this AI coming in, people are starting to content, right? And of course they need to have an expert in the loop and add the creativity and the fun and the specialization, the expertise into it.
And I'm seeing that corporations don't have a good content management plan. So for an example, in life sciences, you may have something called something in clinical research and it gets to commercialization and it changes name. And that's one thing in English when you're trying to communicate, but you add that in across languages and it gets really confusing.
So one of the services that we're offering is kind of a communication strategy. We haven't packaged [00:37:00] it clearly enough, but we're coming into companies and we're talking to them. Okay. Where are your different touch points along the buyer's journey, which is everything from prospect to, you know, service delivery across all parts of the organization.
And so in that you have to understand content, marketing, creation, technology. Are any of your students coming out with this kind of broader perspective of communications and leveraging language and content creation that we can groom them to be consultants in this area?
Lynne Bowker: So, it is something that has not been well addressed, I would say, in translation training curricula in the past because we have students for three or four years, and we have so much to teach them, and so it's getting even harder to, to do that, but, I think we have to, and I'm really glad that you have kind of put that out [00:38:00] there as a genuine need, because it's getting more and more difficult to say that somebody is just a translator. I think we need to broaden our perspective and say I'm a language expert and I can do translation, but I also can do content creation and marketing and bring those things together in a way that someone who's not multilingual can't do.
I don't think we do it well currently but it's a direction that is on the radar now for people that we need to create a more well rounded kind of thing. And actually at my own university right now, we're called the School of Translation and Interpretation, and that we are finding is a bit limiting.
Even though we do include in our translation program, writing and terminology management and, and more than just translation we still call it a BA in translation, it's a school of translation and that sends the message out to the wider public that all we do is translation.
We have to ourselves undergo a sort of rebranding exercise. Either we need [00:39:00] to redefine translation and what that entails to something broader or we need to relabel it as something broader and global communications. Exactly.
Wendy: Which then expands it to, so somebody going in to get a degree in translation has to be fully bilingual, but there's a need for people that may not be fully bilingual, but can understand the whole content management.
Yeah, exactly. Are you teaching your students how to do machine translation with post editing and to use? To do that.
Lynne Bowker: We are. We have a kind of quite a wide range of technologies that our students work with. So we have the translation memories that you mentioned earlier and the terminology management package that's often integrated with the translation memory.
Machine translation can also be housed in that same environment. So you can preference if you like your translation memory, but in cases where you don't
find a match in your translation memory, you can then turn to machine translation for a proposal or [00:40:00] something. So we've got technologies working together but sometimes people want to work sort of solely with machine translation or sometimes all they get is the output of machine translation that someone else has produced and then they're asked, as you say, to post edit or to kind of improve on that and fix it up. So we do work with that as well. Yes.
Wendy: Yeah. And then the struggle there is, is they've got the language, they've got the skills to use the technology, but do they have the subject matter expertise?
Lynne Bowker: Yeah. And that, again, is something that we don't handle well at the bachelor's level. We're seeing not just here at, at my university, but across the education sector more broadly.
More and more programs that are offering a professional master's where they anticipate that someone will come and want to kind of change gears from maybe they're a lawyer, they have a degree in law, but now they want to be a legal translator or they're an engineer and now they want to be a technical translator.
And so this master's level training assumes that people [00:41:00] have a subject specialization already. So not sort of trying to take someone who has a B. A. in French or a B. A. in Literature and turn them into a translator, but someone who has a domain expertise. And those are, the types of translators who continue to see a need for human knowledge.
Because if you remember how I said how those data-driven translation tools work, they don't work well if they don't have enough examples. And the more specialized your subject matter, the fewer examples you can have. So this is an area, again, this is where it comes down to like figuring out what are the smart applications for machine translation and what are the less smart applications.
Specialized text is not a smart application at the moment. And that's an area where people still have an edge, a big edge, I would say like somebody who has a law degree and, and also knows how to translate like, wow, that's a powerhouse person right there.
Wendy: Right, right. Yeah, that is.
And those are the type of people that we're hiring [00:42:00] and they're still struggling with accepting the machine translation as the start, because sometimes it takes them longer to edit that, than it does just to create it.
Lynne Bowker: Yes, and that's I guess where that project triangle comes back into play.
It's sort of like if it doesn't make sense if it's not faster, maybe that's not the way to approach it.
Wendy: Dr. Bowker, this is a fabulous conversation. I really, really appreciate it. You have so many insights, so much information to share. I'm kind of tempted to sign up to get the degree at your...
Lynne Bowker: Well, you could at least read the book.
Wendy: I will definitely read the book. And then if you want any help with the whole consulting, the bigger picture of the global communications, I would love to get into that because I have a vision of it as to what I'd like people... Yeah. Wonderful. All right.
Lynne Bowker: Well, I look forward to a future, a continued [00:43:00] conversation with you then.
Wendy: Good. And I have not pre-prepared you for this question, but I always like to ask people at the end of an episode, what your favorite word is. Favorite foreign word, and foreign can be defined as any language.
Lynne Bowker: I love the word in French, épouvantail, just because it rolls off the tongue in a fun way, and it means scarecrow.
It's not like a useful word, but it's a fun word. I like it. I don't know why, I just like it. Say it again. épouvantail.
Wendy: That's a great one. That is an actually great one. Scarecrow in French. Who would have thought? Alright, where can people reach you if they want to?
Lynne Bowker: You can reach me by email at L B O W K E R at U O T T A W A dot [00:44:00] C A.
That's at the University of Ottawa in Canada.
Wendy: Okay. Well, thank you so much. This has been fabulous. And like I said listeners will put her book link, her LinkedIn, her email on the show notes. So if you want to reach out to Dr. Bowker, you can. Thank you. Any final words of recommendation for people?
Lynne Bowker: Just not to be afraid of technology, but not to be overly trusting of it either, right? We just got to learn to find balance. Yeah.
Wendy: Okay, excellent. So if you listened to this episode and enjoyed it, please pass it on to somebody else that is playing around with AI or is curious about machine translation because this is The expert on it.
And if you have any interest in exploring , hiring a consultant for your global communications, surely reach out to us at Rapport International as we're putting together programs for this, we'd be happy to help do a free call with you. [00:45:00] So that's RapportTranslations. com and I hope you enjoy this episode and we'll talk to you next time.
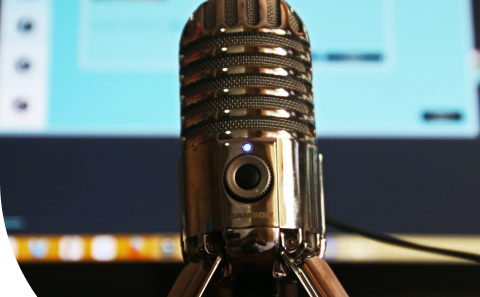
Are you a global marketing professional with stories to share?
Popular Posts
Popular industry news, interviews, technologies, and resources.
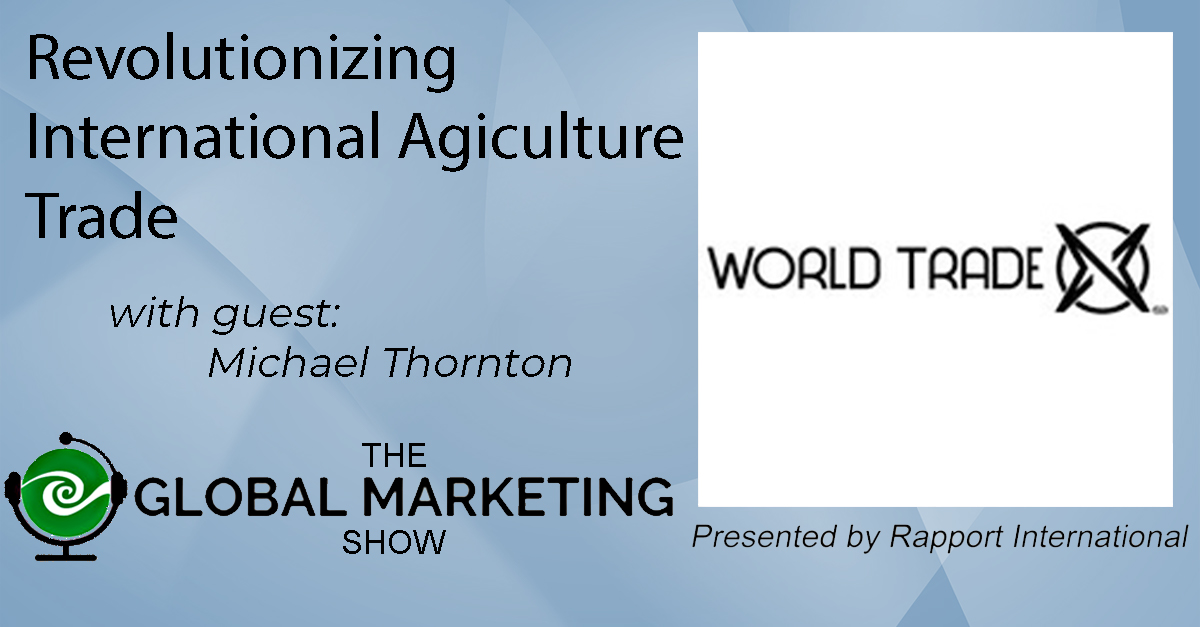
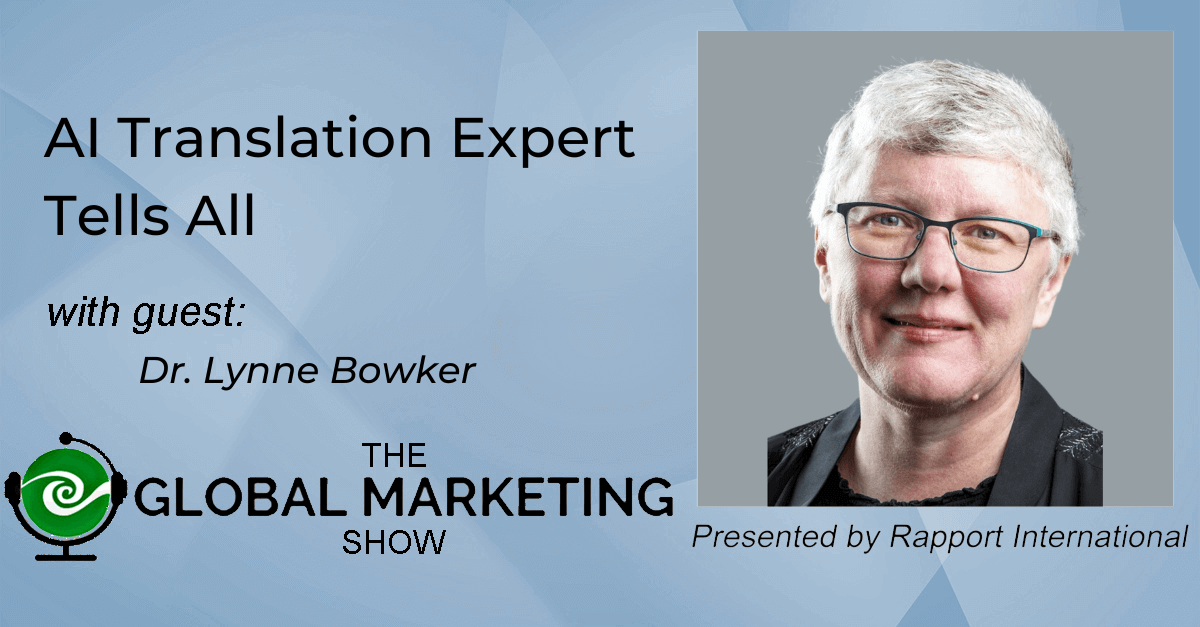
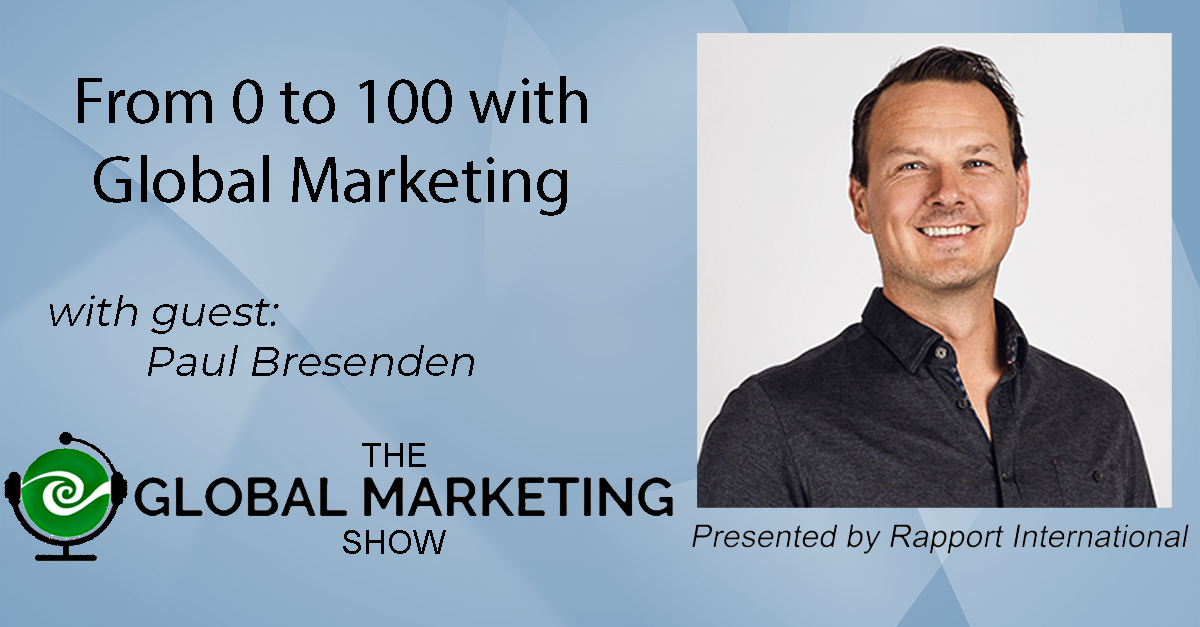
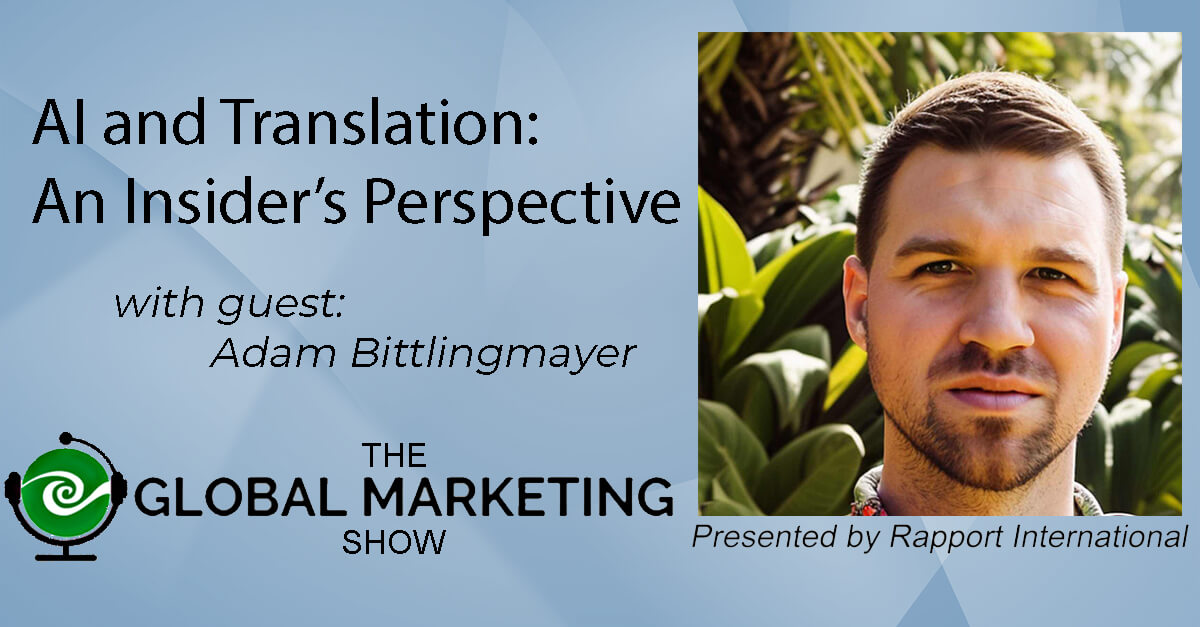
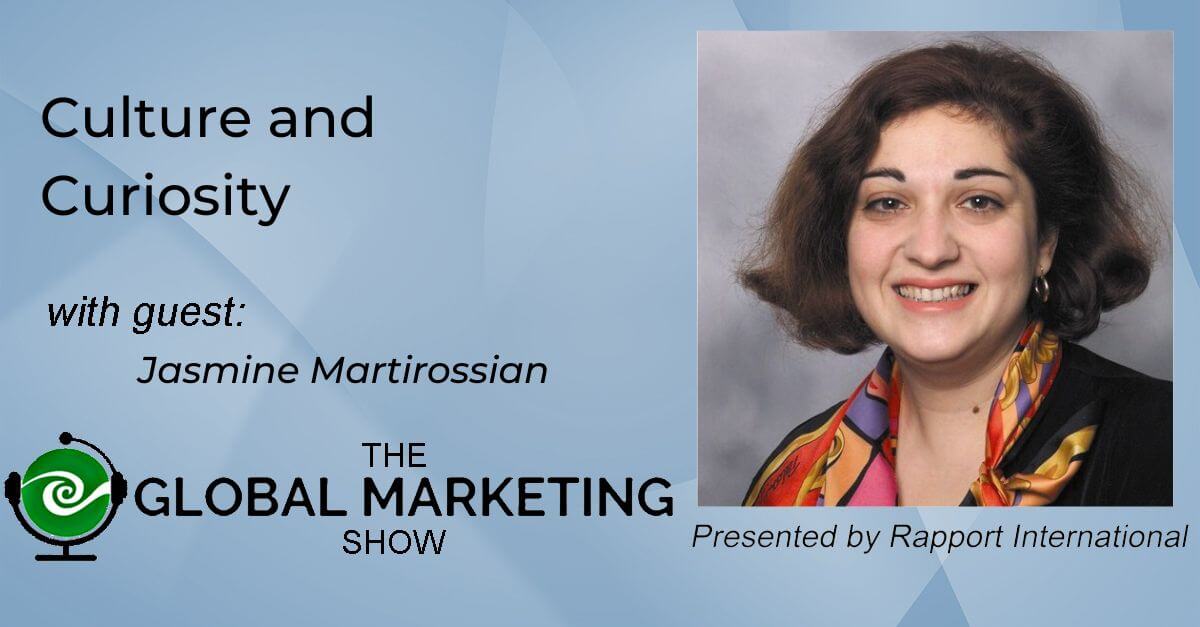
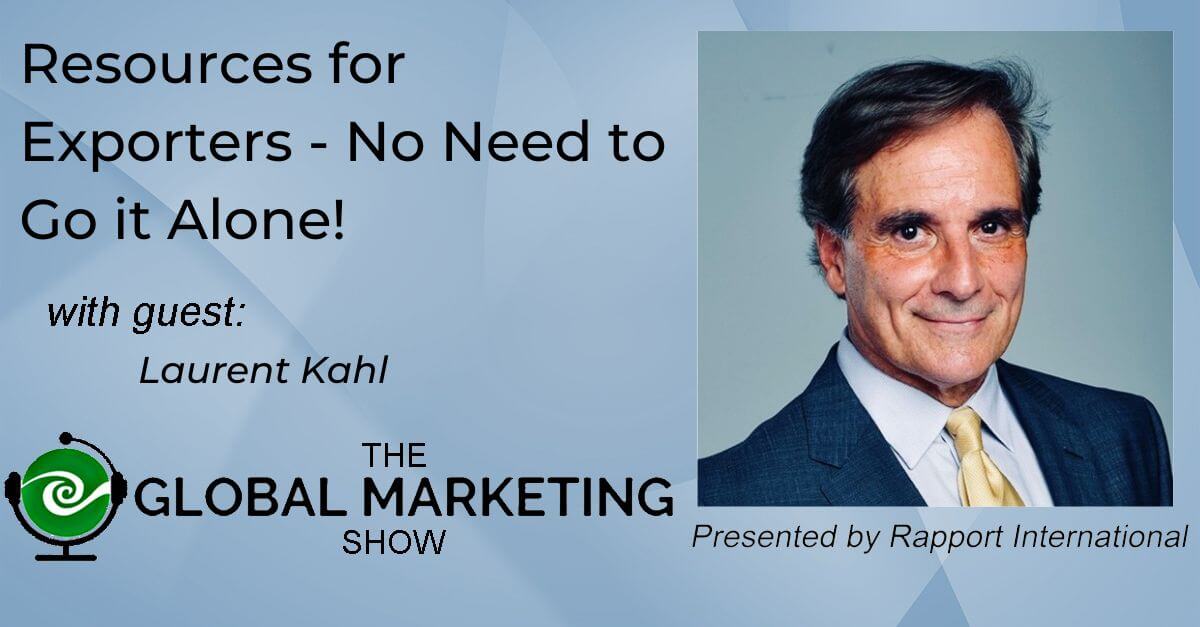
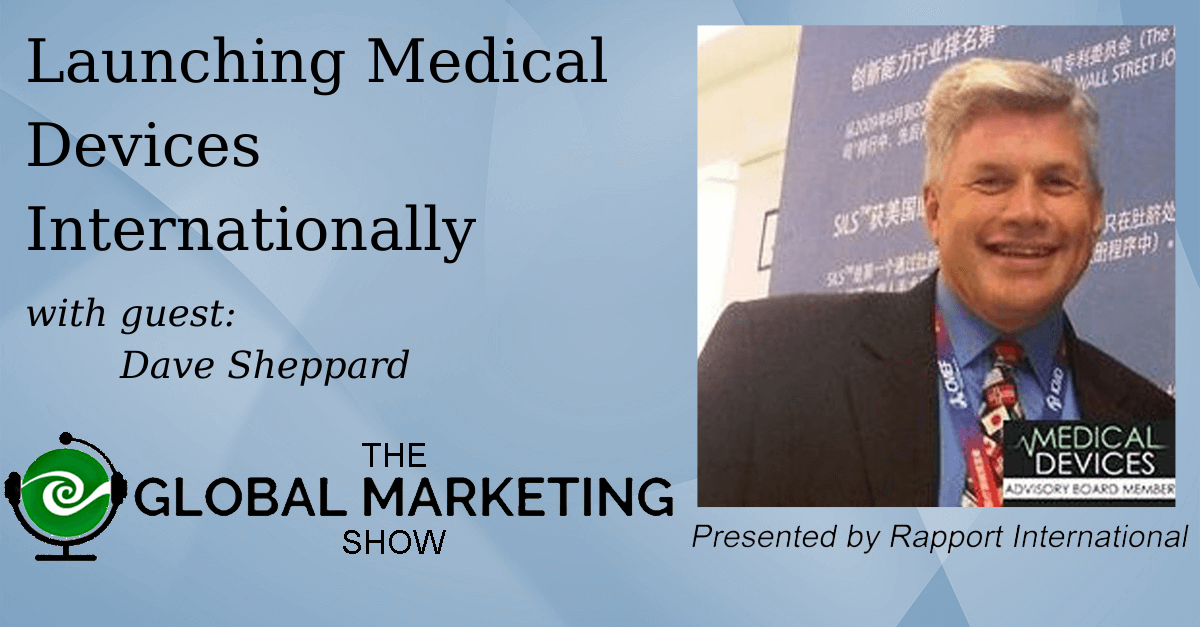
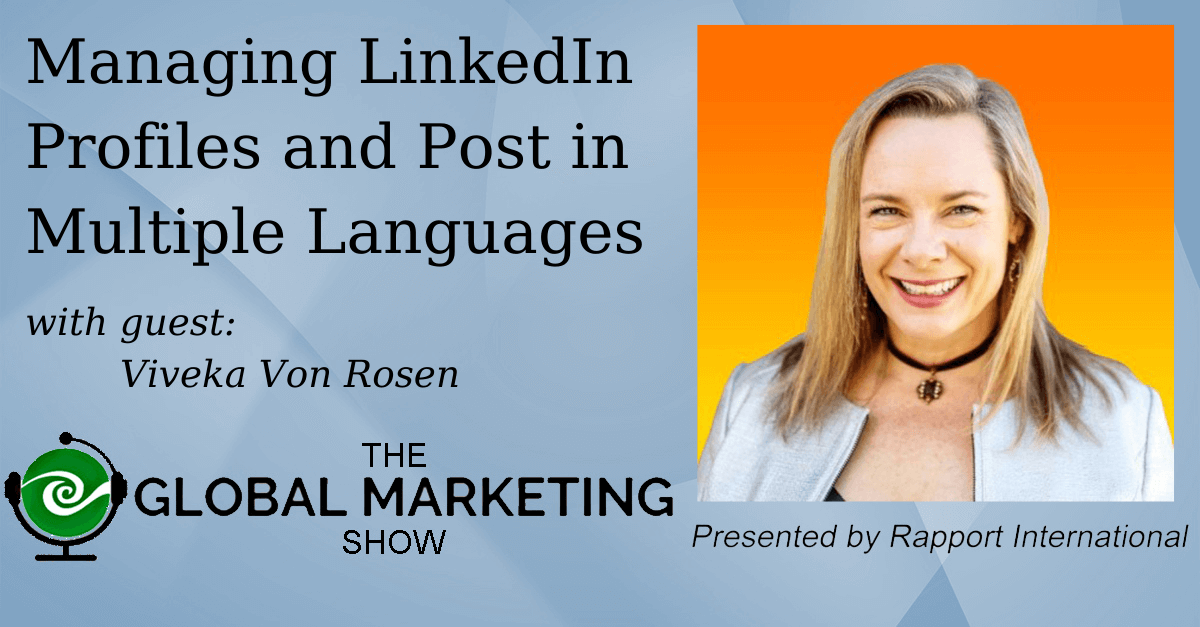
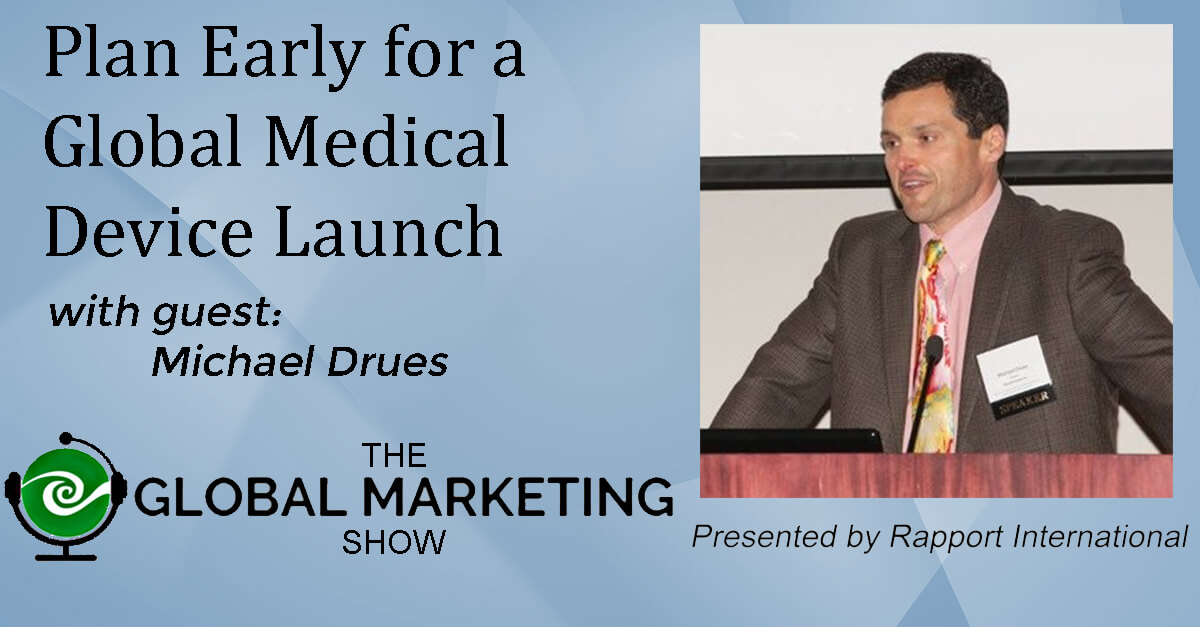
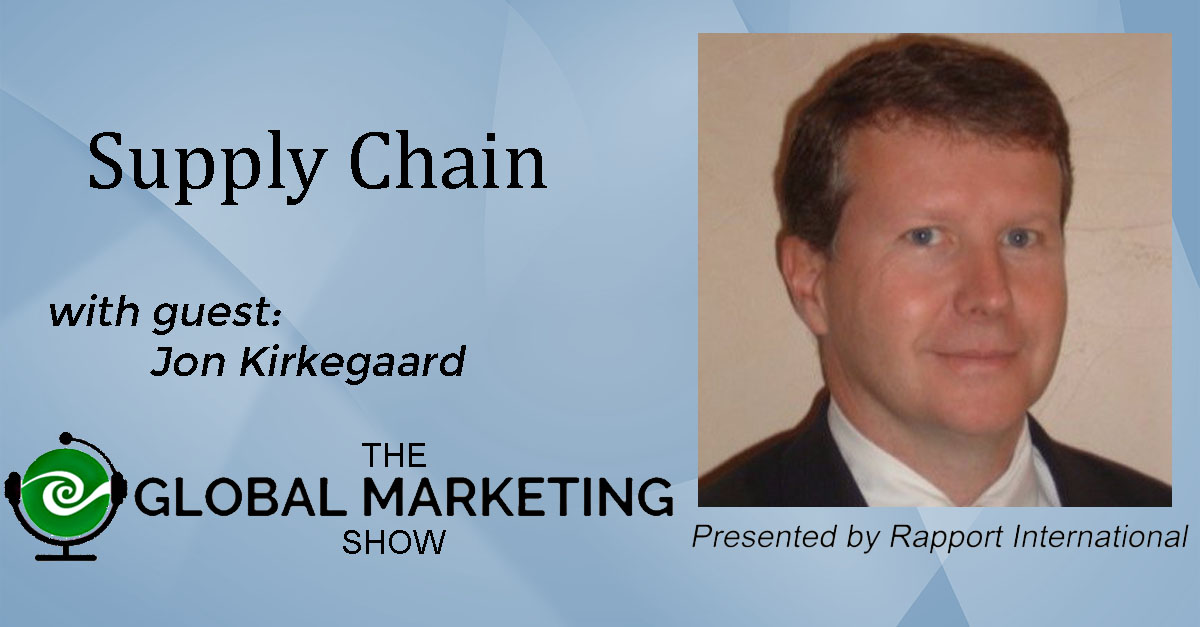